Data-driven organizations use data to:
Measure past performance.
Set measurable targets to track future performance.
Although using data is an essential input into decision-making and reviewing feature performance, there are potential pitfalls to data-driven decision-making that you want to avoid.
Let's take a look at some of these on both the organizational and product management level.
Some organizational pitfalls include:
Measuring the wrong things.
Not measuring something important.
Incentives with unintended consequences.
Managers using data selectively.
Some product pitfalls include:
No explanation for observed data.
False causality.
Local optimization.
The "ex post" dilemma (which refers to only being able to draw conclusions "after the fact").
Organizational behavior
1. Measuring the wrong things
It is incumbent upon the product manager to define appropriate things to measure that define product success. However, doing this effectively is harder than it sounds.
For example, if you measure the total number of users, that number is only ever going to increase. If you were to look at a graph of "total number of users," that graph would show a line going "up and to the right." It tells you very little.
The eBay example
A great example of this is the online auction site eBay.
One early eBay competitor, the business-to-business platform FreeMarkets (from 1998-2002) had more volume (auctions) than eBay yet made tiny revenues in comparison.
What metric might be suitable for eBay to measure? Take a moment and try to guess!
It turns out that the "percentage of transactions resulting in a sale" was the key metric. FreeMarkets had more auctions, yet was less financially successful. When a large percentage of transactions resulted in a sale, buyers felt it was "worth their while" to bid, and sellers felt it was "worth their while" to list products. It wasn't just the available number of products for sale. It was how likely buyers and sellers felt that their time (bidding or listing) was well-spent.
The fighter plane example
During World War II, fighter planes that sustained gunfire were analyzed to see which areas to strengthen. Adding steel reinforcements made the plane slower and heavier. The goal became to add the right amount of reinforcements in the right areas, which was where they were hit the most often.
Efforts to reinforce the planes did not lessen the number of casualties. When a team of statisticians examined the problem, they realized that engines weren't reinforced. That was because planes that were shot in the engine didn't usually come back to base and were not part of the previous analysis.
2. Not measuring something important
The consequence of not measuring something important is that the product manager may be making decisions that damage their product and not realize it.
There may be a trade-off between making additional revenue on a website and making customers happy. This is especially true if the revenue requires showing more ads (typically regarded as an "interruption" by customers). In this example, measuring revenue alone would not tell the full story - you would need also to measure customer engagement to know if you were doing a good job or not. Making additional revenue at the cost of losing half of your customer base would not be a success.
Former United States Secretary of Defense Donald Rumsfeld once said:
As we know, there are known knowns; there are things we know we know. We also know there are known unknowns; that is to say we know there are some things we do not know. But there are also unknown unknowns – the ones we don't know we don't know. And if one looks throughout the history of our country and other free countries, it is the latter category that tend to be the difficult ones.
This is the challenge for all product managers - to be open to the phenomena that you don't know that you don't know. You can do this by digging deeper when the data points you to unexpected behavior in your product. For example, if the number of people returning products doubled last week, you should call those people to ask why. In this way, you use quantitative data to drive you to seek qualitative explanations.
Ignoring all qualitative factors is known as the McNamara paradox. Robert McNamara was the United States Secretary of Defense during the Vietnam War. His only metric for success was to count the enemy's fatalities thus ignoring other quantitative factors such as public opinion.
3. The effect of unintended consequences
When seeking to reward top performance, managers may decide to provide benefits (extra salary, bonuses or promotions) to employees who reach certain numerical targets.
The problem is that those targets may be achievable - but at a cost. For example, salespeople may be able to reach targets by making promises that the company can't keep.
A support team may be told to solve as many cases as possible within five days. They may end up ignoring cases that can't be fixed within the time period.
Management intended to encourage the team to reduce wait time. However, it consequently caused difficult cases to be ignored. (this certainly was not management's intention!)
The cobra example
Due to the high fatality rates from cobra bites in India during the 19th century, the British Empire paid for every cobra skin brought to them. Although the intention was to reduce the snake population while giving an income to the hunters, the incentives actually had the opposite effect.
People actually began to farm cobras so they could increase the number of skins they could sell. Production of cobras became an industry. The British Empire realized that their incentives were having the wrong impact and they stopped paying. The cobra farmers then released their snakes. The end result of this well-intended program was an increased population of cobras!
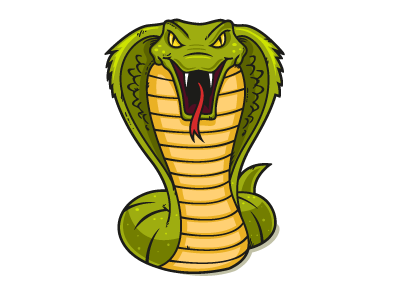
The high jump example
Sergei Bubka was an Olympic gold medal pole vault champion from Ukraine. During his time, Nike offered a $100k reward each time the world record was broken in this sport. This incentive led Bubka to break his world record by the smallest amount possible so that he could break it again in the future.
Bubka broke his own world record 14 times, which was not what Nike had intended. For publicity reasons, they wanted to see the record smashed rather than broken in small increments. Their reward caused an unintended consequence.
4. Using data selectively
The advertising tycoon David Ogilvy once said:
Most people use analytics the way a drunk uses a lamppost, for support rather than illumination.
If you try to find some data that supports your cause, you will likely be able to find something.
Product behavior
1. Not finding out why
Data can indicate when and where user behavior has changed. However, it doesn't explain the cause of the behavior change. In order to understand that, you have to dig further and add qualitative understanding to quantitative data.
If the data tells you that shoe sales on your site have dramatically fallen, is because the website is broken somehow? Or slow? Or because the pricing is incorrect? Or deliveries have been very slow in recent weeks? You need to find out.
2. False causality
It is vital to understand the distinction between correlation and causation.
Correlation - When the incidence of event A means that the likelihood of event B is increased then A and B are correlated.
Causation - When the incidence of event A means that event B will happen, then A causes B and there is causation between A and B.
For example, let's say you increased prices on your site and the number of sales dropped. Was this because you increased prices?
What if I told you that the security certificate on your site was expired and your visitors saw "site cannot be trusted" messages. Now, which of the two events caused the drop in sales?
Just because two events happen at the same time doesn't mean that one event caused the other. Consider the correlation between the number of people who drown in swimming pools, and the number of films that Nicolas Cage appeared in. Does one cause the other? Obviously not! But, when one number is high, the other tends to be high.
3. The local optimization conundrum
When you try to optimize a metric such as revenue, you can sometimes think that you have reached the limit of what is possible.
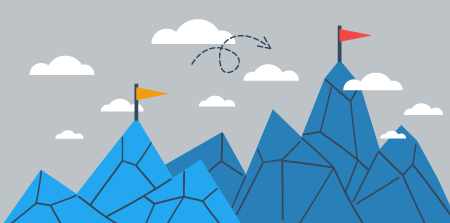
However, sometimes this can be because you are making 'small' changes and the maximum possible revenue (the orange flag) is the most you can achieve without making "big" changes.
Sometimes changing the color of a button, or the image size on a website can lead to a certain optimization of metrics (conversion, revenue, etc.) To get really big improvements (to get to the red flag), you need to change more than the color of a button. You may have to make a fundamental change like offering free shipping or a six-month guarantee.
When optimizing, always ask yourself if you have achieved a local maximum (orange flag), or an absolute maximum (red flag).
4. The "ex post" dilemma
"Ex post" means "after the event." Sometimes you can only know something after the event, based on your knowledge of the past.
Before Steve Jobs released the iPhone, there was no data to state that iPhones would be a success (people didn't even know what an iPhone was). How could Steve Jobs have used data in this scenario? The answer is that maybe other proxy data can tell you something useful. Perhaps the releases of other phones could be interesting data for him?
The other answer is that sometimes a product manager cannot have data for everything. Perhaps the iPhone was so unique that no true data could exist for predicting its success in advance of its launch.
Harvard Professor Youngme Moon sums this up nicely:
If we pay attention to things that we can measure, we will only pay attention to the things that are easily measurable. And in the process we will miss a lot.
Summary
Effective organizations should use data as an essential input into decision-making and reviewing company performance.
However, there are some pitfalls that you want to avoid when using data to drive decisions:
Some organizational pitfalls include:
Measuring the wrong things.
Not measuring something important.
Incentives with unintended consequences.
Managers using data selectively.
Some product pitfalls include:
No explanation for observed data
False causality
Local optimization
The 'ex post' dilemma
Additional resources
A great article showing the cherry picking of economic data on economicshelp.org.
More spurious correlation graphs.
Jordan Ellenberg tells the story of the fighter planes.