Supervised machine learning is a subset of machine learning, which is a discipline within the field of artificial intelligence. This picture puts the contents of this course into context:
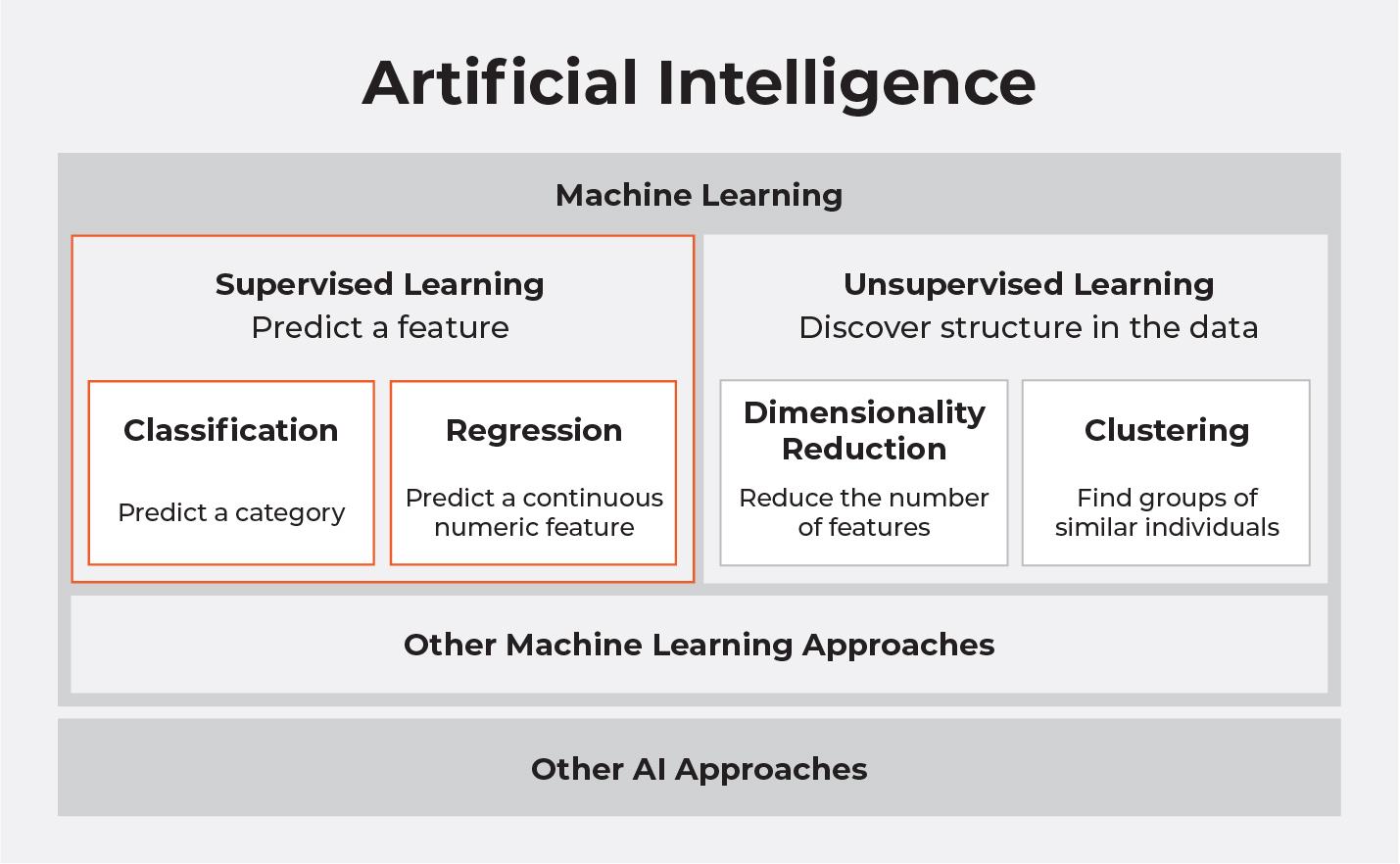
In the field of artificial intelligence (AI), we build models that represent and solve a particular problem. Machine learning semi-automates the creation of these models for particular AI tasks. For example, you can automate the building of a model that forecasts the weather.
Supervised learning uses examples of the thing you are trying to predict like pictures of rabbits to build a model that can find other pictures of rabbits.
Unsupervised learning builds models without examples to discover structure within data.
We will focus on two types of supervised learning tasks: classification, for predicting a category, and regression, for predicting a continuous numeric feature.
Here are some real-world classification problems:
Filtering out spam emails.
Determining which patients suffer from a particular disease.
Recognizing hand-written letters and numbers.
Here are some real-world regression problems:
Estimating property prices.
Forecasting stock prices.
Predicting sales for a retail store.
Some multiple algorithms and techniques can be used within each approach, and through skill, experimentation, and lots of practice, the data scientist can craft useful machine learning models.
Your models will be built using algorithms, which are computational techniques or rules used to solve a problem in a particular way. An algorithm is like a recipe that provides a finite list of instructions to perform a task. You will also need a dataset to build models, which is a sample of data. Generally, the rows contain data points (or sample points), which are the individual examples in your data. The columns represent features, which are the characteristics of the data points.
Humans Do Supervised Learning Too!
Take a look at these shapes:
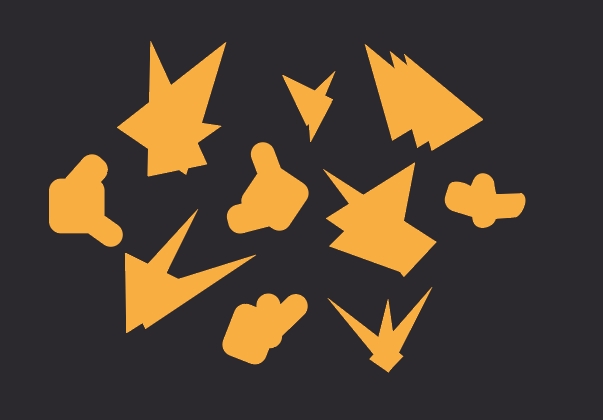
What if I told you that some are called Twipis, and some are called Pondles? Could you tell me which ones are which?
Probably not, although you might take a lucky guess!
What if I helped a bit, and said these are Twipis:
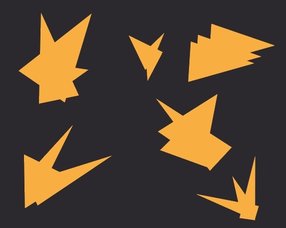
These are Pondles:
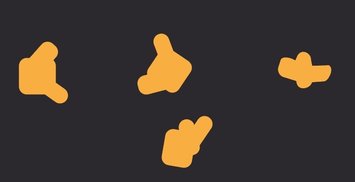
Does that help?
You should now be able to classify all the shapes as Twipis or Pondles. You may have come up with some rules, based on the examples I gave. Perhaps your rules looked something like this:
IF shape has spiky edges, it’s a Twipi.
ELSE IF shape has round edges, it’s a Pondle,
What if I introduced another shape, Yootups.
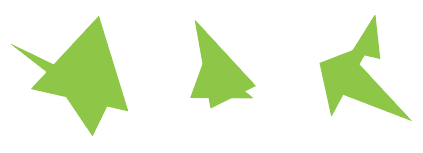
Would your rules change?
And if I said the following were also Pondles:
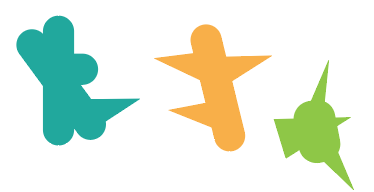
Would your rules change again?
Your rules may look something like this:
IF shape has at least one rounded edge, it’s a Pondle.
ELSE IF shape has only spiky edges and is orange, it’s a Twipi.
ELSE IF shape has only spiky edges and is green, it’s a Yootup.
What Did We Just Do?
What you have here are three classes:
Twipi
, Pondle
,and Yootup
And two features:
Edge shape
and Color
When I told you the name of the shapes (Twipis, Pondles, and Yootups), I gave you pre-labeled samples. From that, you built a set of rules, or in other words, a model.
You can use the model to classify the pre-labeled samples, as well as the other samples I provided. What’s more, given some new sample shapes, I’m sure you would be reasonably confident that you could classify most of them correctly as Twipis, Pondles, or Youtups. I’m sure you are a little concerned I might spring something on you that is slightly ambiguous such as this:
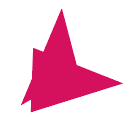
This process of learning by example is quite natural and what you are fundamentally doing when you build supervised machine learning models.
Using Decision Trees
The rules for the above model can be expressed in a diagram called a decision tree. It looks like this:
We just created a classification task, which is a model (determined set of rules) that would predict in which class a sample point belonged. Decision trees are just one of the algorithms you will encounter when building machine learning models.
When a decision tree is used to classify a sample, one sample point is taken at a time and tested against the rules from top to bottom. Think of it as pouring the sample into the top of the decision tree and, based on the rules, the sample points flow to a single class at the bottom:
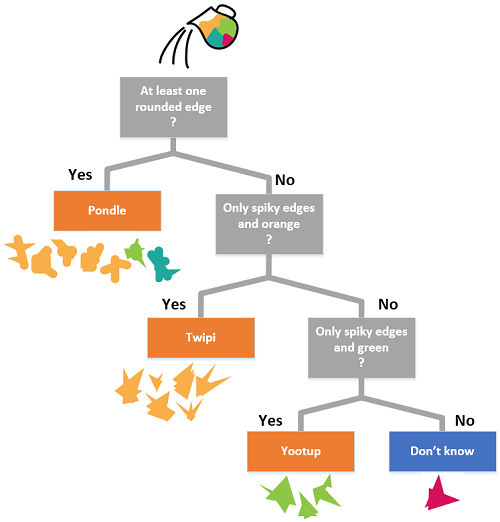
Recap
Supervised learning tasks are a subset of machine learning.
Classification is a supervised learning task.
Take an algorithm, and feed it some pre-labeled samples, to create a model.
Pre-labeled samples consist of input features and a single output or target feature.
Use the model to classify some un-labeled samples.
A decision tree is just one of the possible algorithms you could use.